Burooj Ghani
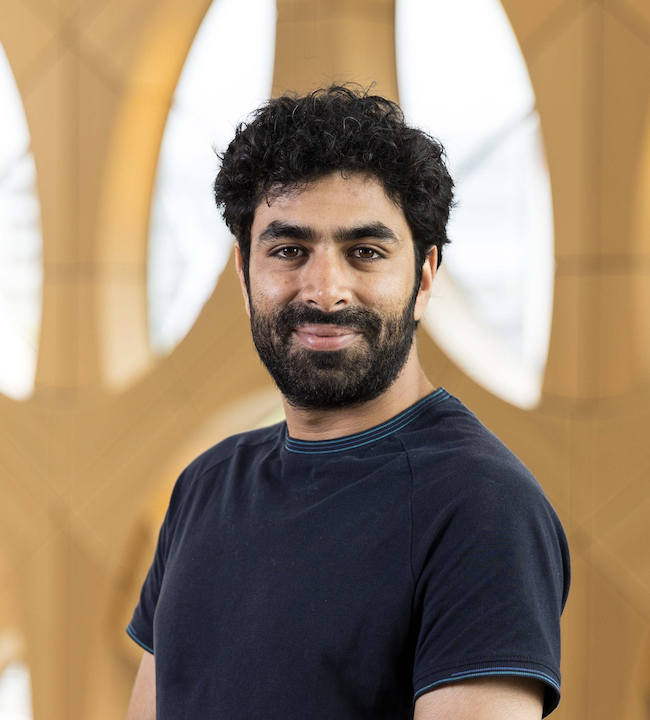
My research focuses on developing robust machine learning methods for biodiversity monitoring, with a particular emphasis on sound-based approaches. I aim at creating scalable, field-deployable solutions for detecting and classifying animal vocalizations, enabling real-time and high-throughput ecological assessments. My broader vision is to contribute to the development of multimodal and multi-scale frameworks that integrate diverse data sources for a more holistic understanding of ecosystems. I obtained a master’s and a PhD in Computer Science from the University of Göttingen in Germany, where I ventured into bioacoustics, focusing on bird species classification, the impact of species selection on classification outcomes, and algorithmic analysis of intra-species song variations.
My current work aims to bridge AI and ecological conservation by advancing computational tools that can be effectively applied in real-world biodiversity monitoring. This has the potential to greatly benefit wildlife experts, conservation biologists and ecologists by providing automated tools for long-term environmental monitoring. The central focus of my current work lies in processing extensive datasets of environmental sounds, enabling the extraction of valuable insights into the hidden world of animal communication. In nature, while many animals are visually elusive, their vocalizations offer a wealth of information about their habitats, seasonal changes, and interactions. My goal is to create automated processes that accelerate and streamline the analysis, ultimately contributing to the protection and conservation of our planet’s diverse species and ecosystems.
news
Mar 13, 2025 | I’ll be co-chairing a symposium titled: “General-purpose Deep Learning: Tapping into the Power of Pretrained Neural Networks” at the International Bioacoustics Conference (IBAC) taking place in Kerteminde, Denmark, from September 7-12, 2025. |
---|---|
Oct 16, 2024 | The birdsong recognition algorithm AvesEcho, which I developed at Naturalis, was added to Xeno-canto, the world’s biggest animal sound repository. The ML model is also the first one to be hosted on the forum. Read more here. The inference model is available in this GitLab repository. |
Sep 25, 2024 | New preprint out! in which we explore different transfer learning methods, pre-trained models, and dataset characteristics to understand the effect on birdsong classification performance, especially out-of-distribution performance. |
Apr 1, 2024 | Just launched the 2024 edition of the Few-shot bioacoustic event detection task within the DCASE challenge. |
Dec 21, 2023 | Our paper is out in Nature Scientific Reports, in which we investigate pre-trained deep learning classifiers for downstream bioacoustics classification tasks and report global birdsong embeddings enable superior transfer learning. |
Dec 17, 2023 | Drew Priebe’s thesis titled: “Efficient speech detection in environmental audio using acoustic recognition and model distillation”, which I had the pleasure of co-supervising alongside Dan Stowell, has resulted in a manuscript. |
Sep 28, 2023 | Will be organising a symposium on “Computational and machine learning methods” at IBAC2023 in Hokkaido, Japan later this year. |
Sep 22, 2023 | Presented my work on bird sound classification at the MAMBO project meeting in Malta. |
Aug 25, 2022 | Starting work as a Postdoctoral Fellow in AI & Biodiversity at the Naturalis Biodiversity Center in Leiden, the Netherlands beginning Jan 2023. I will be working closely with Dan Stowell to develop audio recognition algorithms to identify a variety of European taxa. |
Mar 25, 2022 | Will be heading to the K. Lisa Yang Center for Conservation Bioacoustics at the Cornell Lab of Ornithology, Cornell University, Ithaca, USA for a 4 months-long research stint. |
in the media
Nov 2, 2024 | Nature Today: A birdsong AI joins the world’s biggest bird sound forum |
---|---|
Jul 6, 2024 | Nature: Interpreting the call of the wild with AI |
Jun 30, 2024 | Nature Today: Zeshonderd uur sprinkhaanmuziek online |